About the Workshop
The CVPR 2020 Workshop on Autonomous Driving (WAD) aims to gather researchers and engineers from academia and industry to discuss the latest advances in perception for autonomous driving. In this one-day workshop, we will have regular paper presentations, invited speakers, and technical benchmark challenges to present the current state of the art, as well as the limitations and future directions for computer vision in autonomous driving, arguably the most promising application of computer vision and AI in general. The previous chapters of the workshop at CVPR attracted hundreds of researchers to attend. This year, multiple industry sponsors also join our organizing efforts to push its success to a new level.
Day Event
Speakers
Participants
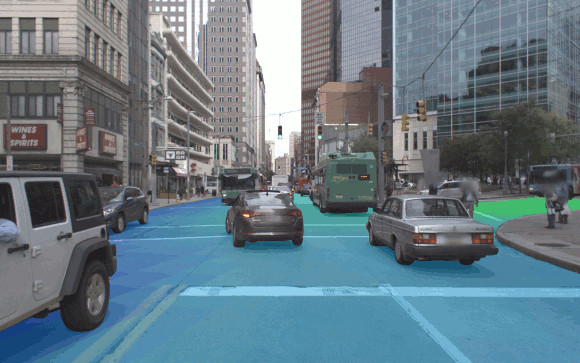
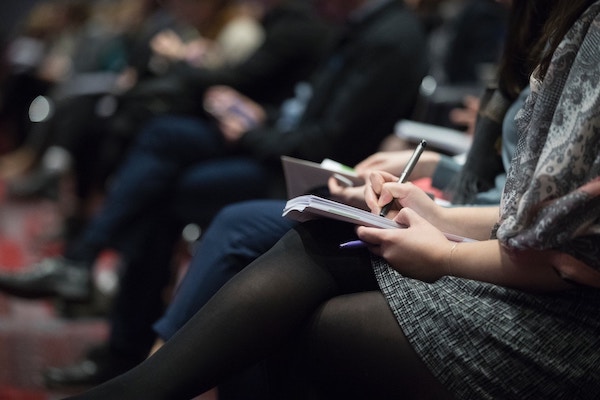
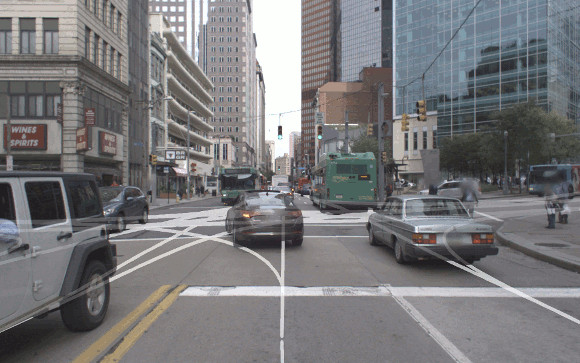
Paper Submission
We solicit paper submissions on novel methods and application scenarios of CV for Autonomous vehicles. We accept papers on a variety of topics, including autonomous navigation and exploration, ADAS, UAV, deep learning, calibration, SLAM, etc.. Papers will be peer reviewed under double-blind policy and the submission deadline is 20th March 2020. Accepted papers will be presented at the poster session, some as orals and one paper will be awarded as the best paper.
Challenge Track
We host a challenge to understand the current status of computer vision algorithms in solving the environmental perception problems for autonomous driving. We have prepared a number of large scale datasets with fine annotation, collected and annotated by Berkeley Deep Driving Consortium and others. Based on the datasets, we have define a set of four realistic problems and encourage new algorithms and pipelines to be invented for autonomous driving).
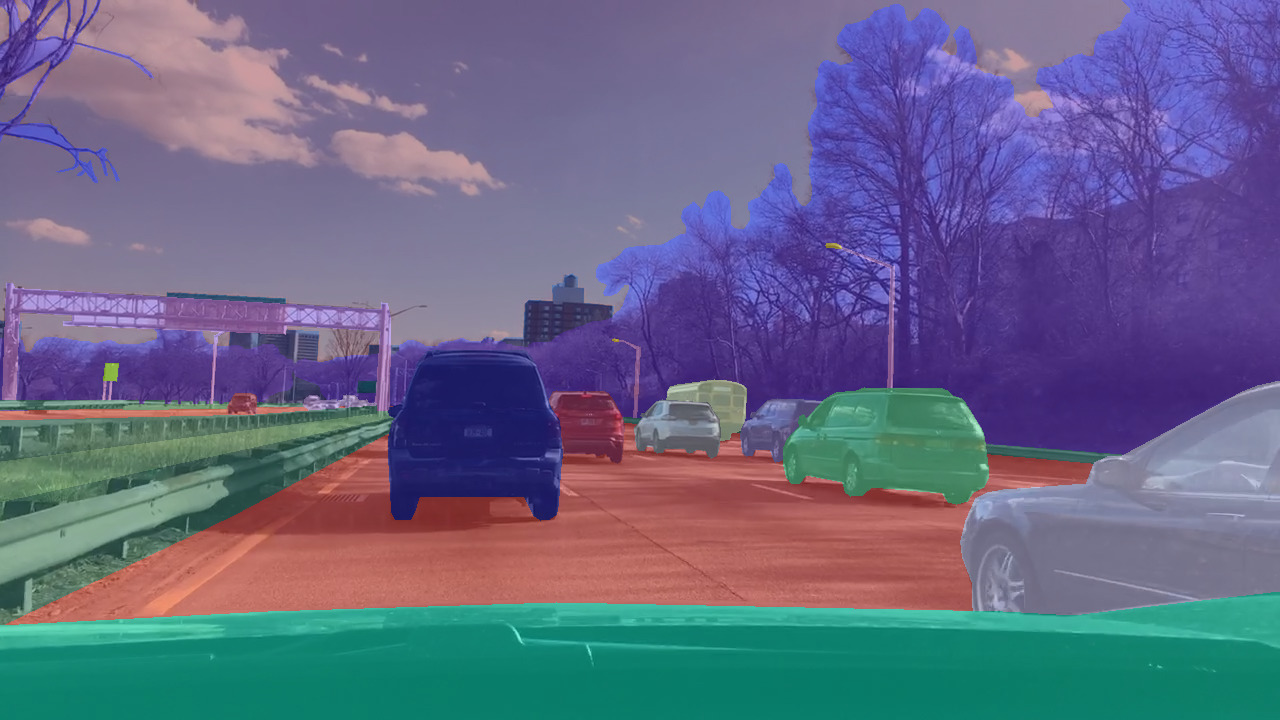
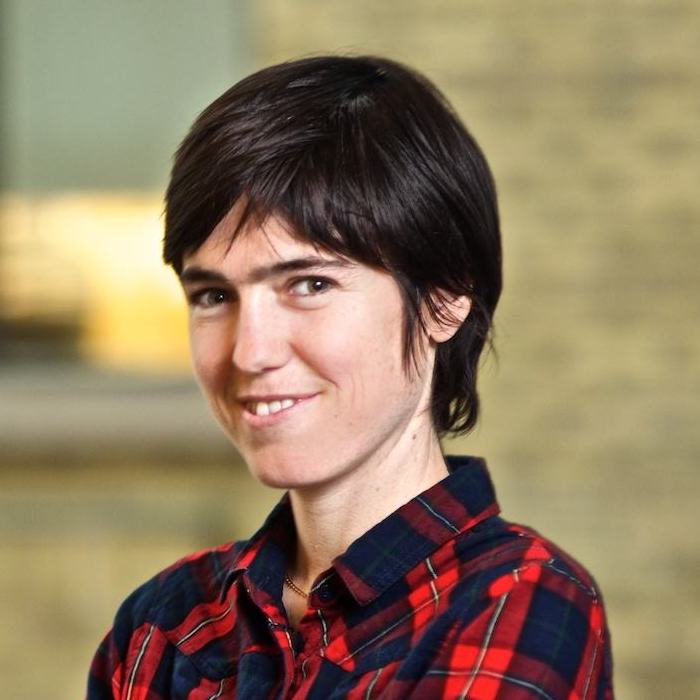
Raquel Urtasun – V2VNet: Vehicle-To-Vehicle Communication for Self-driving
Absrtract: While a world densely populated with self-driving vehicles (SDVs) might seem futuristic, these vehicles will soon be the norm. They will provide safer, cheaper and less congested transportation for everyone, everywhere. A core component of self-driving is perception and motion forecasting: from sensor data, the SDV needs to identify other agents and forecast their futures. However, state-of-the-art perception and motion forecasting algorithms still have difficulty with heavily occluded or far away actors due to sparse sensor observations. Failing to detect and predict the intention of these hard-to-see actors might have catastrophic consequences in safety critical situations.
But what if SDVs could transmit information and utilize the information received from nearby vehicles? This could allow us to see farther and behind occlusions, and detect objects earlier, allowing for safer and more comfortable maneuvers.
In this talk I’ll showcase V2VNet, the first sophisticated AI-based vehicle-to-vehicle communication approach for self-driving. I’ll show that to achieve the best compromise of perception and prediction (P&P) performance while also satisfying existing hardware transmission bandwidth capabilities, we should send compressed intermediate representations of each vehicle’s P&P neural network. V2VNet then utilizes a spatially aware graph neural network (GNN) to aggregate the information received from nearby SDVs. Our experiments in a large-scale V2V dataset show that we can reduce perception errors by 70%.
The talk will be hosted on youtube.
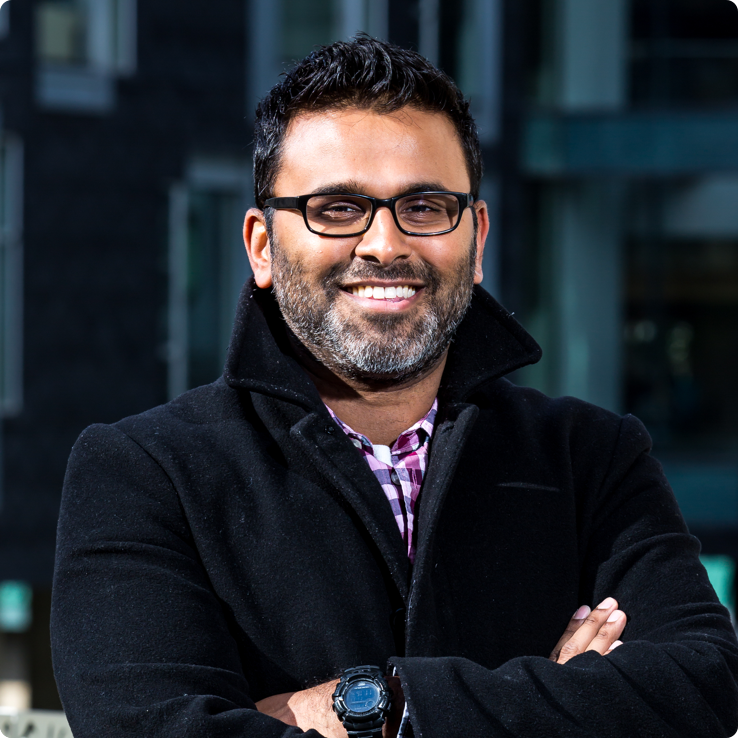
Deva Ramanan – Embodied perception in-the-wild
Absrtract: Computer vision is undergoing a period of rapid progress, rekindling the relationship between perception, action, and cognition. Such connections may be best practically explored in the context of autonomous robotics. In this talk, I will discuss perceptual understanding tasks motivated by embodied robots "in-the-wild", focusing on the illustrative case of autonomous vehicles. I will argue that many challenges that surface are not well-explored in contemporary computer vision. These include streaming computation with bounded resources, generalization via spatiotemporal grouping, online behavioral forecasting, and self-aware processing that can recognize anomalous out-of-sample data. I will conclude with a description of open challenges for embodied perception in-the-wild.
You can find the talk on the CVPR workshop homepage.
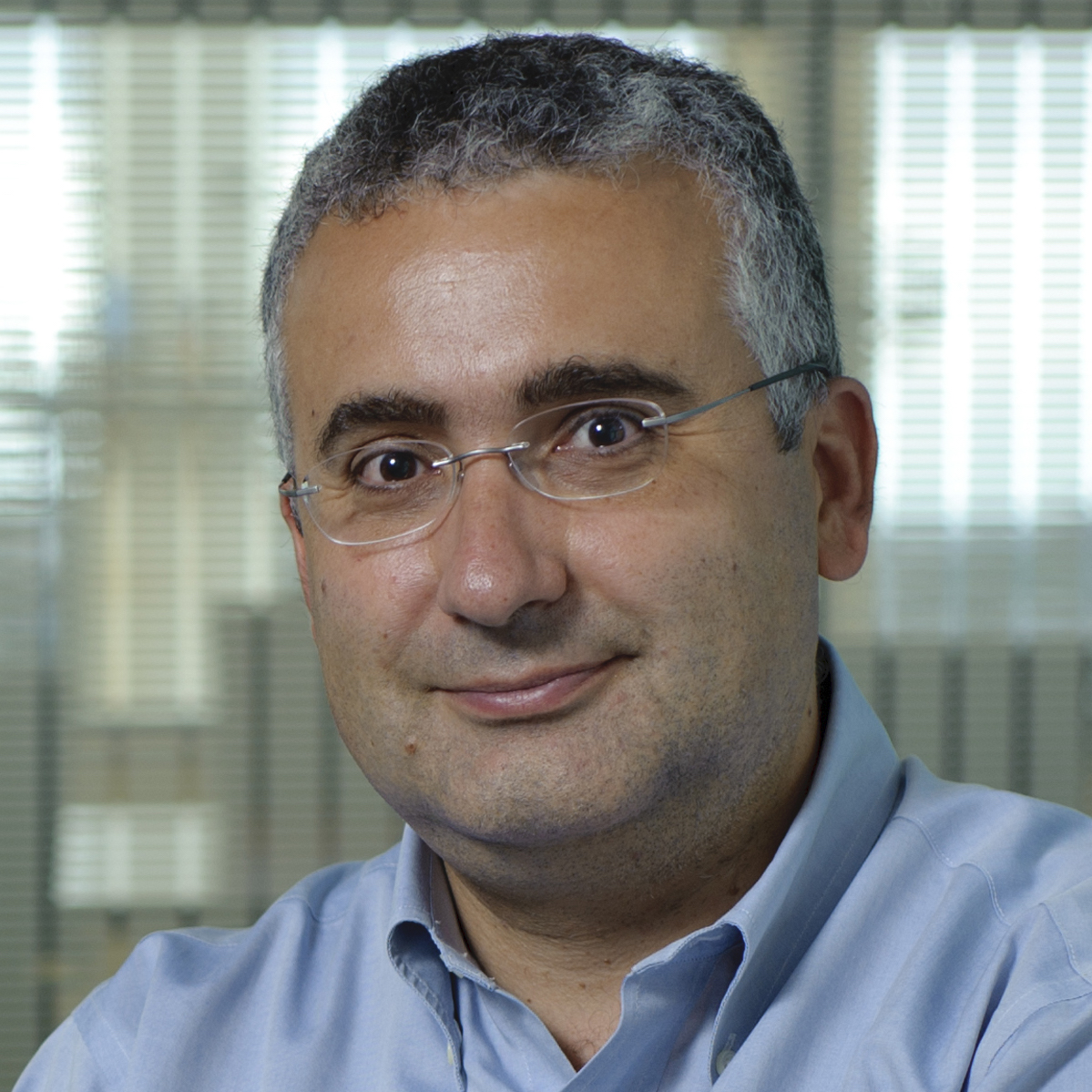
Emilio Frazzoli – Multi-modal Wormhole Learning
Absrtract: Self-driving cars currently on the road are equipped with dozens of sensors of several types (lidar, radar, sonar, cameras, . . . ). All of this existing and emerging complexity opens up many interesting questions regarding how to deal with multi-modal perception and learning. The recently developed technique of “wormhole learning” shows that even temporary access to a different sensor with complementary invariance characteristics can be used to enlarge the operating domain of an existing object detector without the use of additional training data. For example, an RGB object detector trained with daytime data can be updated to function at night time by using a “wormhole” jump through a different modality that is more illumination invariant, such as an IR camera. It turns out that having an additional sensor improves performance, even if you subsequently lose it. In this work we show that the wormhole learning concept goes beyond vision sensors only. Namely, we explain why (and when) it works in structured geometric setups, in more general cases via information theory, and experimentally with RBG, IR and event-based cameras for object detections. The geometric analysis hinges on groups' theory to model invariances and domain shifts, allowing us to gain also visual intuition for the simplest cases of points in the plane. Information theory allows us to answer more general questions such as "how much information can be gained?" with statistical bounds. Finally, we show experimentally that wormhole learning works also for sensors that are radically different, such as RGB cameras and event-based neuromorphic sensors.
You can find the talk on the CVPR workshop homepage.
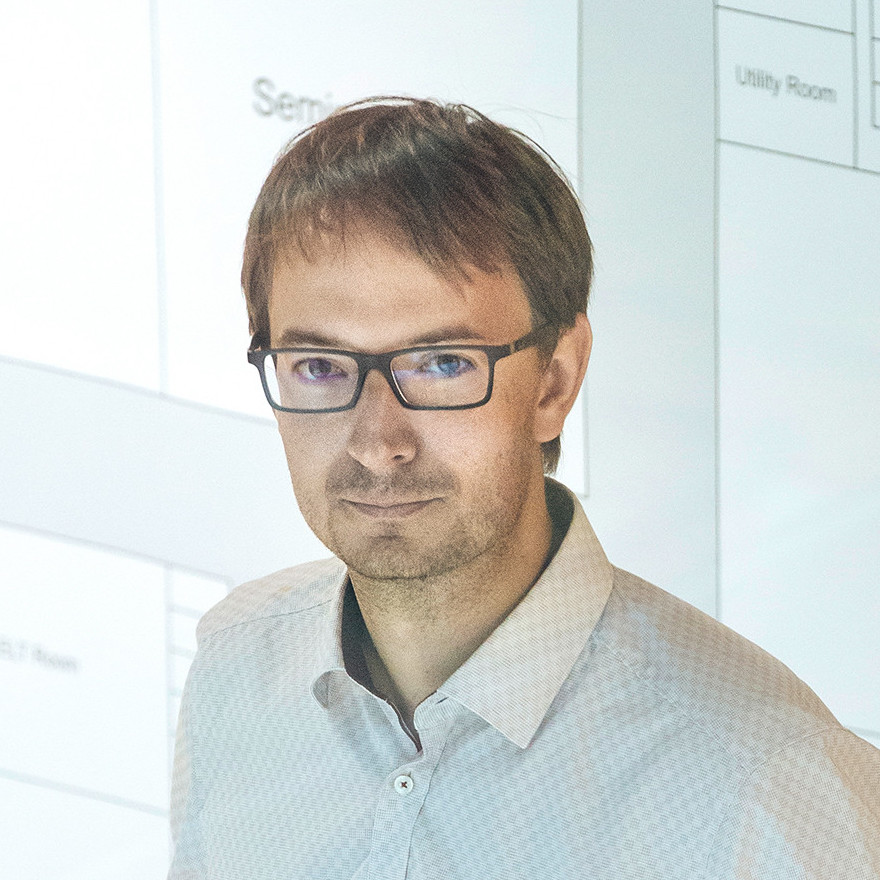
Andreas Geiger – Learning Robust Driving Policies
I will present two recent results on learning robust driving policies that lead to state-of-the-art performance in the CARLA simulator. To generalize across diverse conditions, humans leverage multiple types of situation-specific reasoning and learning strategies. Motivated by this observation, I will first present a new framework for learning a situational driving policy that effectively captures reasoning under varying types of scenarios and leads to 98% success rate on the CARLA driving benchmark as well as state-of-the-art performance on a newly introduced generalization benchmark. In the second part of my talk, I will discuss the problem of covariate shift in imitation learning. I will demonstrate that the majority of data aggregation techniques for addressing this problem have poor generalization performance, and present a novel approach with empirically better generalization performance. The key idea is to sample critical states from the collected on-policy data based on the utility they provide to the learned policy and to incorporate a replay buffer which progressively focuses on the high uncertainty regions of the policy's state distribution. The proposed approach is evaluated on the CARLA NoCrash benchmark, focusing on the most challenging driving scenarios with dense pedestrian and vehicle traffic, achieving 87% of the expert performance while also reducing the collision rate by an order of magnitude without the use of any additional modality, auxiliary tasks, architectural modifications or reward from the environment.
The talk will be hosted on youtube.
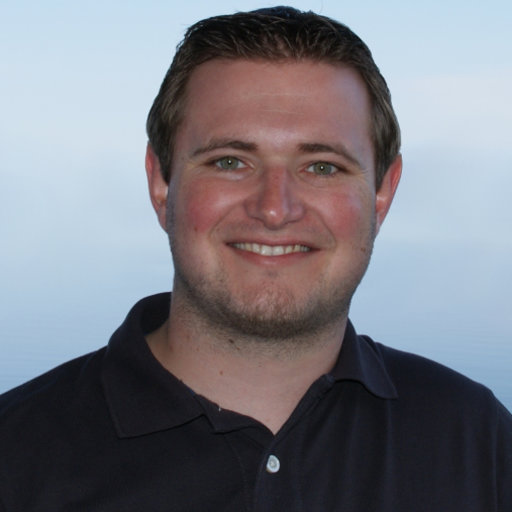
Andreas Wendel – A Day in the Life of a Self-Driving Truck
Absrtract: In this talk, Kodiak Robotics’ VP of Engineering, Andreas Wendel, gives insights into the development of self-driving semi-trucks. Starting from the shop where the vehicles are outfitted with sensors and actuators, the journey along the highway explores the technological differences between trucks and passenger vehicles, perception and motion planning challenges, and business considerations.
The talk will be hosted on youtube.
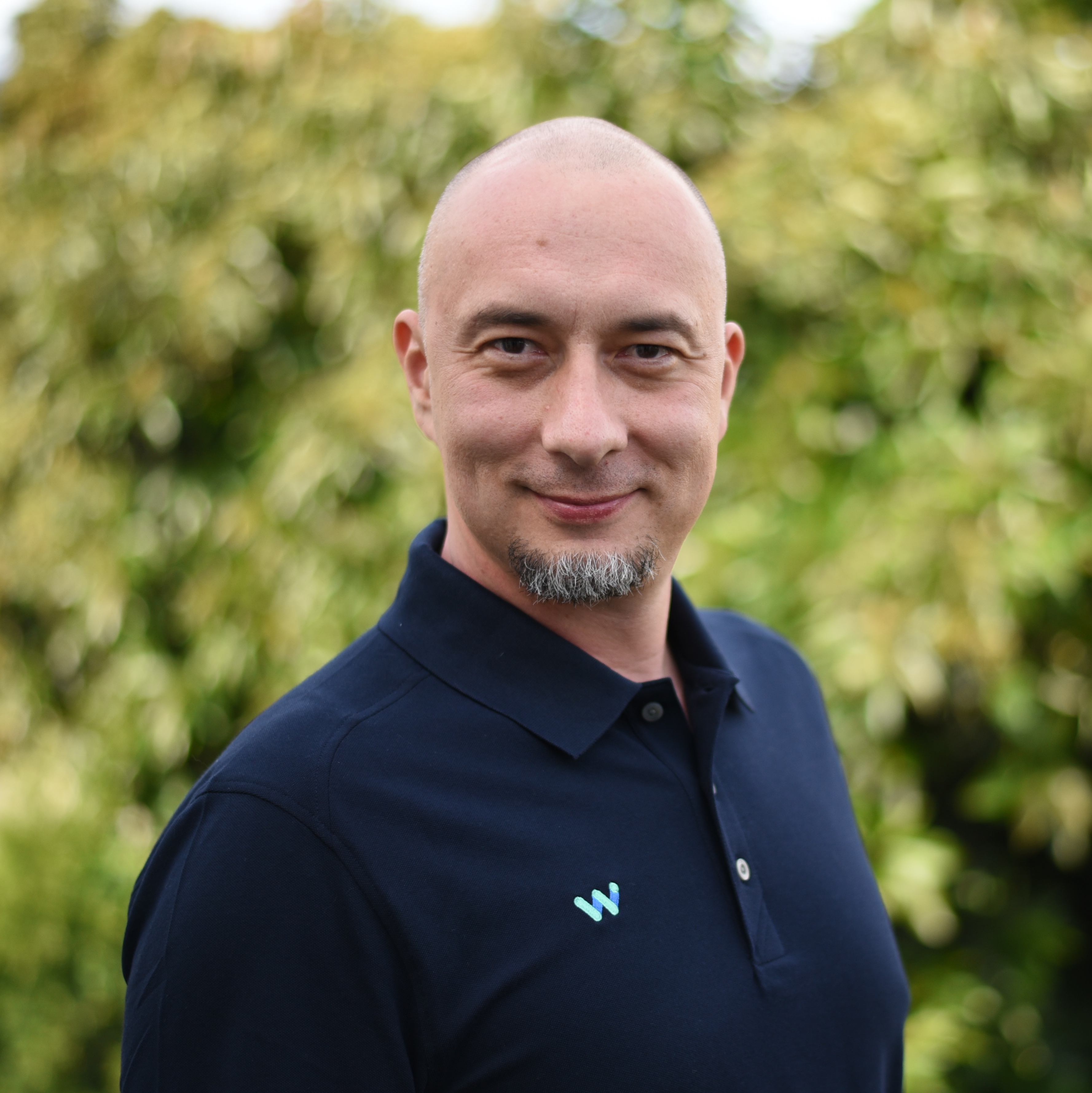
Drago Anguelov – Machine Learning for Autonomous Driving at Scale
Absrtract: Machine learning is key to developing a self-driving stack that can scale to a diverse set of environments without requiring exhaustive manual labeling or expert tuning. In this talk, I will describe some of the recent modeling work at Waymo that aims to capture better the inherent structure in the autonomous driving domain. I will give an update on the Waymo Open Dataset and the recently completed Challenges and highlight some of our recent work on self-supervision of perception models, as well as data-driven approaches for sensor simulation.
The talk will be hosted on youtube.
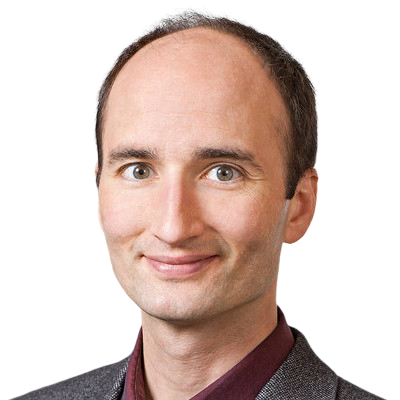
Daniel Cremers – Deep Direct Visual SLAM
I will discuss recent achievements on boosting the performance of direct methods for visual simultaneous localization and mapping using deep networks.
You can find the talk on the CVPR workshop homepage.
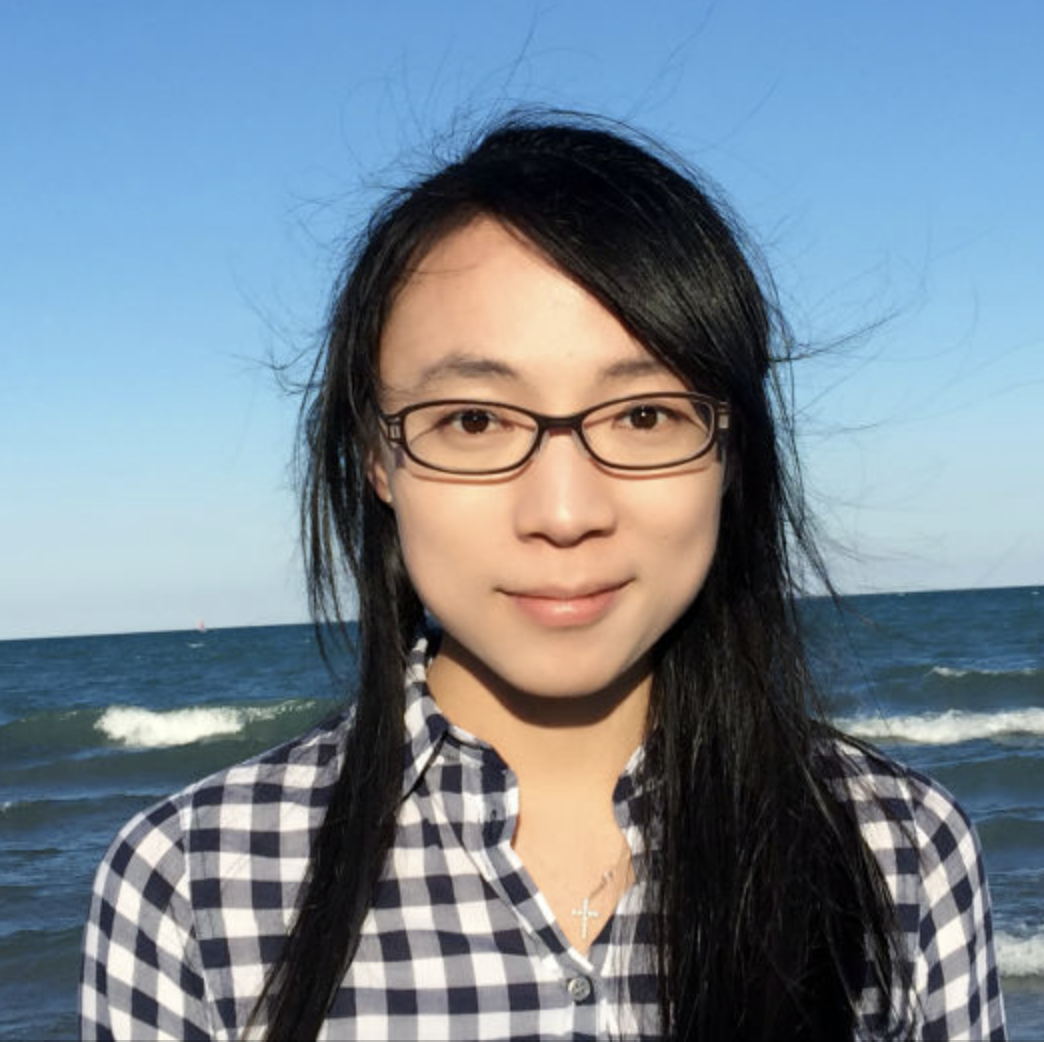
Bo Li – Secure Learning in Adversarial Autonomous Driving Environments
Absrtract: Advances in machine learning have led to rapid and widespread deployment of software-based inference and decision making, resulting in various applications such as data analytics, autonomous systems, and security diagnostics. Current machine learning systems, however, assume that training and test data follow the same, or similar, distributions, and do not consider active adversaries manipulating either distribution. Recent work has demonstrated that motivated adversaries can circumvent anomaly detection or other machine learning models at test time through evasion attacks, or can inject well-crafted malicious instances into training data to induce errors in inference time through poisoning attacks. In this talk, I will present the research about physical attacks against different perceptron sensors of autonomous driving vehicles including the multi-sensor fusion systems, and also discuss the potential defensive approaches such as the "sensing-reasoning" pipeline and principles towards developing real-world robust learning systems.Advances in machine learning have led to rapid and widespread deployment of software-based inference and decision making, resulting in various applications such as data analytics, autonomous systems, and security diagnostics. Current machine learning systems, however, assume that training and test data follow the same, or similar, distributions, and do not consider active adversaries manipulating either distribution. Recent work has demonstrated that motivated adversaries can circumvent anomaly detection or other machine learning models at test time through evasion attacks, or can inject well-crafted malicious instances into training data to induce errors in inference time through poisoning attacks. In this talk, I will present the research about physical attacks against different perceptron sensors of autonomous driving vehicles including the multi-sensor fusion systems, and also discuss the potential defensive approaches such as the "sensing-reasoning" pipeline and principles towards developing real-world robust learning systems.
The talk will be hosted on youtube.
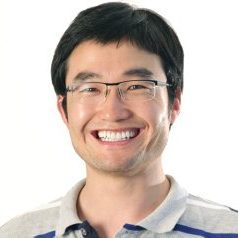
Dengxin Dai – Towards All-Season and Social Self-Driving Cars
Absrtract: In this talk, I will speak about two important challenges that must be overcome to turn the current ‘somewhat automated’ into full automation and to let the society better accept self-driving cars. The first one is named all-weather self-driving. Adverse weather/illumination conditions create visibility problems for the sensors that power automated systems. The ability to robustly cope with ``bad'' weather and lighting conditions is absolutely essential for self-driving cars. In this talk, I will first present our recent work on semantic driving scene understanding under adverse weather/illumination conditions. This involves a diverse range of learning paradigms including domain adaptation, synthetic data, map-guided learning, and knowledge distillation. The second challenge is social self-driving. However autonomously a car could act, there will always be a need to let the human occupants interact with it, leading to a high-level mixed control. In order to better fit into the social context, the car also needs to learn to interact with other road users by using multimodal cues such as audio signals, gaze, and gestures. For the second half of the talk, I will show our recent work on human-to-car dialogue based on natural speech and semantic auditory perception with binaural sounds.
You can find the talk on the CVPR workshop homepage.
- 9:00 am
-
Argoverse Challenge Link to CVPR Event
- 9:30 am
-
BDD100K Multi-Object Tracking Challenge Link to CVPR Event
- 10.00 am
-
Panel discussion and Q&A invited speakers With: Andreas Geiger, Andreas Wendel, Bo Li, Daniel Cremers, Dengxin Dai, Deva Ramanan, Drago Anguelov, Emilio Frazzoli, Raquel Urtasun
Link to CVPR Event - 11:00 am
-
Q&A paper authorsWith: TBD
Link to CVPR Event - 9:00 pm
-
Argoverse ChallengeLink to CVPR Event
- 9:30 pm
-
BDD100K Multi-Object Tracking Challenge Link to CVPR Event
- 10.00 pm
- 11:00 pm
-
Q&A paper authorsWith: TBD
Link to CVPR Event
Important Dates
- Workshop paper submission deadline:
March 14th 2020March 20th 2020Notification to authors:9th April 202015th April 2020Camera ready deadline: 19th April 2020Topics Covered
Topics of the papers include but are not limited to:
- Autonomous navigation and explorationVision based advanced driving assistance systems, driver monitoring and advanced interfacesVision systems for unmanned aerial and underwater vehiclesDeep Learning, machine learning, and image analysis techniques in vehicle technologyPerformance evaluation of vehicular applicationsOn-board calibration of acquisition systems (e.g., cameras, radars, lidars)3D reconstruction and understandingVision based localization (e.g., place recognition, visual odometry, SLAM)
Presentation Guidelines
All accepted papers will be presented as posters. The guidelines for the posters are the same as at the main conference.Submission Guidelines
- We solicit short papers on autonomous vehicle topicsSubmitted manuscript should follow the CVPR 2019 paper templateThe page limit is 8 pages (excluding references)We accept dual submissions, but the manuscript must contain substantial original contents not submitted to any other conference, workshop or journalSubmissions will be rejected without review if they:
- contain more than 8 pages (excluding references)violate the double-blind policy or violate the dual-submission policyThe accepted papers will be linked at the workshop webpage and also in the main conference proceedings if the authors agreePapers will be peer reviewed under double-blind policy, and must be submitted online through the CMT submission system at: https://cmt3.research.microsoft.com/WAD2020
Accepted PapersSMOKE: Single-Stage Monocular 3D Object Detection via Keypoint Estimation
Authors: Zechen Liu, Zizhang Wu and Roland Toth
Abstract: Estimating 3D orientation and translation of objects is essential for infrastructure-less autonomous navigation and driving. In case of monocular vision, successful methods have been mainly based on two ingredients: (i) a network generating 2D region proposals, (ii) a R-CNN structure predicting 3D object pose by utilizing the acquired regions of interest. We argue that the 2D detection network is redundant and introduces non-negligible noise for 3D detection. Hence, we propose a novel 3D object detection method, named SMOKE, in this paper that predicts a 3D bounding box for each detected object by combining a single keypoint estimate with regressed 3D variables. As a second contribution, we propose a multi-step disentangling approach for constructing the 3D bounding box, which significantly improves both training convergence and detection accuracy. In contrast to previous 3D detection techniques, our method does not require complicated pre/post-processing, extra data, and a refinement stage. Despite of its structural simplicity, our proposed SMOKE network outperforms all existing monocular 3D detection methods on the KITTI dataset, giving the best state-of-the-art result on both 3D object detection and Bird's eye view evaluation. The code will be made publicly available.
The presentation can be found here: cvpr20.com/event/smoke-single-stage-monocular-3d-object-detection-via-keypoint-estimation/
The paper can be found here: openaccess@thecvfWasserstein Loss based Deep Object Detection
Authors: Xiaofeng Liu, Jane You, Yutao Ren, Risheng Liu, Zhenfei Sheng, Xu Han, Yuzhuo Han and Zhongxuan Luo
Abstract: Object detection locates the objects with bounding boxes and identifies their classes, which is valuable in many computer vision applications (e.g. autonomous driving). Most existing deep learning-based methods output a probability vector for instance classification trained with the one-hot label. However, the limitation of these models lies in attribute perception because they do not take the severity of different misclassifications into consideration. In this paper, we propose a novel method based on the Wasserstein distance called Wasserstein Loss based Model for Object Detection (WLOD). Different from the commonly used distance metric such as cross-entropy (CE), the Wasserstein loss assigns different weights for one sample identified to different classes with different values. Our distance metric is designed by combining the CE or binary cross-entropy (BCE) with Wasserstein distance to learn the detector considering both the discrimination and the seriousness of different misclassifications. The misclassified objects are identified to similar classes with a higher probability to reduce intolerable misclassifications. Finally, the model is tested on the BDD100K and KITTI datasets and reaches state-of-the-art performance.
The presentation can be found here: cvpr20.com/event/wasserstein-loss-based-deep-object-detection/
The paper can be found here: openaccess@thecvfLearning Depth-Guided Convolutions for Monocular 3D Object Detection
Authors: Mingyu Ding, Yuqi Huo, Hongwei Yi, Zhe Wang, Jianping Shi, Zhiwu Lu and Ping Luo
Abstract: 3D object detection from a single image without LiDAR is a challenging task due to the lack of accurate depth information. Conventional 2D convolutions are unsuitable for this task because they fail to capture local object and its scale information, which are vital for 3D object detection. To better represent 3D structure, prior arts typically transform depth maps estimated from 2D images into a pseudo-LiDAR representation, and then apply existing 3D point-cloud based object detectors. However, their results depend heavily on the accuracy of the estimated depth maps, resulting in suboptimal performance. In this work, instead of using pseudo-LiDAR representation, we improve the fundamental 2D fully convolutions by proposing a new local convolutional network (LCN), termed Depth-guided Dynamic-Depthwise-Dilated LCN (D4LCN), where the filters and their receptive fields can be automatically learned from image-based depth maps, making different pixels of different images have different filters. D4LCN overcomes the limitation of conventional 2D convolutions and narrows the gap between image representation and 3D point cloud representation. Extensive experiments show that D4LCN outperforms existing works by large margins. For example, the relative improvement of D4LCN against the state-of-the-art on KITTI is 9.1% in the moderate setting.
The presentation can be found here: cvpr20.com/event/learning-depth-guided-convolutions-for-monocular-3d-object-detection-2/
The paper can be found here: openaccess@thecvfFeudal Steering: Hierarchical Learning for Steering Angle Prediction
Authors: Faith M Johnson and Kristin Dana
Abstract: We consider the challenge of automated steering angle prediction for self driving cars using egocentric road images. In this work, we explore the use of feudal networks, used in hierarchical reinforcement learning (HRL), to devise a vehicle agent to predict steering angles from first person, dash-cam images of the Udacity driving dataset. Our method, Feudal Steering, is inspired by recent work in HRL consisting of a manager network and a worker network that operate on different temporal scales and have different goals. The manager works at a temporal scale that is relatively coarse compared to the worker and has a higher level, task-oriented goal space. Using feudal learning to divide the task into manager and worker sub-networks provides more accurate and robust prediction. Temporal abstraction in driving allows more complex primitives than the steering angle at a single time instance. Composite actions comprise a subroutine or skill that can be re-used throughout the driving sequence. The associated subroutine id is the manager network’s goal, so that the manager seeks to succeed at the high level task (e.g. a sharp right turn, a slight right turn, moving straight in traffic, or moving straight unencumbered by traffic). The steering angle at a particular time instance is the worker network output which is regulated by the manager’s high level task. We demonstrate state-of-the art steering angle prediction results on the Udacity dataset.
The presentation can be found here: cvpr20.com/event/feudal-steering-hierarchical-learning-for-steering-angle-prediction/
The paper can be found here: openaccess@thecvfSelf-supervised Object Motion and Depth Estimation from Video
Authors: Qi Dai, Vaishakh Patil, Simon Hecker, Dengxin Dai, Luc Van Gool and Konrad Schindler
Abstract: We present a self-supervised learning framework to estimate the individual object motion and monocular depth from video. We model the object motion as a 6 degree-of-freedom rigid-body transformation. The instance segmentation mask is leveraged to introduce the information of object. Compared with methods which predict dense optical flow map to model the motion, our approach significantly reduces the number of values to be estimated. Our system eliminates the scale ambiguity of motion prediction through imposing a novel geometric constraint loss term. Experiments on KITTI driving dataset demonstrate our system is capable to capture the object motion without external annotation. Our system outperforms previous self-supervised approaches in terms of 3D scene flow prediction, and contribute to the disparity prediction in dynamic area.
The presentation can be found here: cvpr20.com/event/self-supervised-object-motion-and-depth-estimation-from-video/
The paper can be found here: openaccess@thecvfEnd-to-End Lane Marker Detection via Row-wise Classification
Authors: Seungwoo Yoo, Heeseok Lee, Heesoo Myeong, Sungrack Yun, Hyoungwoo Park, Janghoon Cho and Duckhoon Kim
Abstract: In autonomous driving, detecting reliable and accurate lane marker positions is a crucial yet challenging task. The conventional approaches for the lane marker detection problem perform a pixel-level dense prediction task followed by sophisticated post-processing that is inevitable since lane markers are typically represented by a collection of line segments without thickness. In this paper, we propose a method performing direct lane marker vertex prediction in an end-to-end manner, i.e., without any post-processing step that is required in the pixel-level dense prediction task. Specifically, we translate the lane marker detection problem into a row-wise classification task, which takes advantage of the innate shape of lane markers but, surprisingly, has not been explored well. In order to compactly extract sufficient information about lane markers which spread from the left to the right in an image, we devise a novel layer, inspired by [8], which is utilized to successively compress horizontal components so enables an end-to-end lane marker detection system where the final lane marker positions are sim- ply obtained via argmax operations in testing time. Experimental results demonstrate the effectiveness of the proposed method, which is on par or outperforms the state-of-the-art methods on two popular lane marker detection benchmarks, i.e., TuSimple and CULane.
The presentation can be found here: cvpr20.com/event/end-to-end-lane-marker-detection-via-row-wise-classification/
The paper can be found here: openaccess@thecvfAn Extensible Multi-Sensor Fusion Framework for 3D Imaging
Authors: Talha Ahmad Siddiqui, Rishi Madhok and Matthew O'Toole
Abstract: Many autonomous vehicles rely on an array of sensors for safe navigation, where each sensor captures different visual attributes from the surrounding environment. For example, a single conventional camera captures high-resolution images but no 3D information; a LiDAR provides excellent range information but poor spatial resolution; and a prototype single-photon LiDAR (SP-LiDAR) can provide a dense but noisy representation of the 3D scene. Although the outputs of these sensors vary dramatically (e.g., 2D images, point clouds, 3D volumes), they all derive from the same 3D scene. We propose an extensible sensor fusion framework that (1) lifts the sensor output to volumetric representations of the 3D scene, (2) fuses these volumes together, and (3) processes the resulting volume with a deep neural network to generate a depth (or disparity) map. Although our framework can potentially extend to many types of sensors, we focus on fusing combinations of three imaging systems: monocular/stereo cameras, regular LiDARs, and SP-LiDARs. To train our neural network, we generate a synthetic dataset through CARLA that contains the individual measurements. We also conduct various fusion ablation experiments and evaluate the results of different sensor combinations.
The presentation can be found here: cvpr20.com/event/an-extensible-multi-sensor-fusion-framework-for-3d-imaging/
The paper can be found here: openaccess@thecvfTopometric Imitation Learning For Route Following Under Appearance Change
Authors: Shaojun Cai and Yingjia Wan
Abstract: Traditional navigation models in autonomous driving rely heavily on metric maps, which severely limits their application in large scale environments. In this paper, we introduce a two-level navigation architecture that contains a topological-metric memory structure and a deep image-based controller. The hybrid memory extracts visual features at each location point with a deep convolutional neural network, and stores information about local driving commands at each location point based on metric information estimated from ego-motion information. The topological-metric memory is seamlessly integrated with a conditional imitation learning controller through the navigational commands that drive the vehicle between different vertices without collision. We test the whole system in teach-and-repeat experiments in an urban driving simulator. Results show that after being trained in a separate environment, the system could quickly adapt to novel environments with a single teach trial and follow route successively under various illumination and weather conditions.
The presentation can be found here: cvpr20.com/event/topometric-imitation-learning-for-route-following-under-appearance-change/
The paper can be found here: openaccess@thecvfChallengesWe host challenges to understand the current status of computer vision algorithms in solving the environmental perception problems for autonomous driving. We have prepared a number of large scale datasets with fine annotation, collected and annotated by Berkeley DeepDriving, Argo AI. Based on the datasets, we have defined a set of several realistic problems and encourage new algorithms and pipelines to be invented for autonomous driving.
Challenge 1: Argoverse Motion Forecasting and 3D Tracking Challenge
Our first two challenges are the Argoverse Motion Forecasting and 3D Tracking challenges. Argo AI is offering $5,000 in prizes for Motion Forecasting and 3D tracking competitions on Argoverse. See more details on the Motion Forecasting and 3D Tracking leaderboards. The competition will end on June 10th. Winning methods will be highlighted during the workshop.
Challenge 2: BDD100K Tracking
We are hosting a multi-object tracking challenge based on BDD100K, the largest open driving video dataset as part of the CVPR 2020 workshop on autonomous driving. This is a large-scale tracking challenge under the most diverse driving conditions. Understanding the temporal association of objects within videos is one of the fundamental yet challenging tasks for autonomous driving. The BDD100K MOT dataset provides diverse driving scenarios with complicated occlusions and reappearing patterns, which serves as a great testbed for the reliability of the developed MOT algorithms in real scenes. We provide 2,000 fully annotated 40-second sequences under different weather conditions, time of the day, and scene types. We encourage participants from both academia and industry and the winning teams will be awarded certificates for the memorable achievement. The challenge webpage: https://bdd-data.berkeley.edu/wad-2020.html.
DatasetsBDD100K Dataset from Berkeley DeepDrive
BDD100K dataset is a large collection of 100K driving videos with diverse scene types and weather conditions. Along with the video data, we also released annotation of different levels on 100K keyframes, including image tagging, object detection, instance segmentation, driving area and lane marking. In 2018, the challenges hosted at CVPR 2018 and AI Challenger 2018 based on BDD data attracted hundreds of teams to compete for best object recognition and segmentation algorithms for autonomous driving.
Argoverse by Argo AI
Argoverse is the first large-scale self-driving data collection to include HD maps with geometric and semantic metadata — such as lane centerlines, lane direction, and driveable area. All of the detail we provide makes it possible to develop more accurate perception algorithms, which in turn will enable self-driving vehicles to safely navigate complex city streets.
OrganizersGeneral Chairs
Program Chairs
Reviewers
Agata Mosinska - RetinAI Medical
Ali Armin - Data61
Caglayan Dicle - nuTonomy
Carlos Becker - Pix4D
Chuong Nguyen - Data61
Eduardo Romera - Universidad de Alcala de Henares
Fatemeh Sadat Saleh - Australian National University (ANU)
George Siogkas - Panasonic Automotive Europe
Helge Rhodin - EPFL
Holger Caesar - nuTonomy
Hsun-Hsien Chang - nuTonomy
Isinsu Katircioglu - EPFL
Joachim Hugonot - EPFL
Kailun Yang
Kashyap Chitta - MPI-IS and University of Tuebingen
Luis Herranz - Computer Vision Center
Mårten Wadenbäck - Chalmers University of Technology and the University of Gothenburg
Mateusz Kozinski -EPFL
Miaomiao Liu - Australian National University
Mohammad Sadegh Aliakbarian - Australian National University
Pablo Márquez Neila - University of Bern
Prof. Dr. Riad I. Hammoud - BAE Systems
Shaodi You University of Amsterdam
Shuai Zheng - VAITL
Shuxuan Guo - EPFL
Sina Samangooei - Five AI Ltd.
Sourabh Vora -nuTonomy
Thomas Probst - ETH Zurich
Timo Rehfeld - Mercedes-Benz R&D
Venice Liong - nuTonomy
Victor Constantin - EPFL
Wei Wang EPFL -
Xiangyu Chen - Shanghai Jiao Tong University
Xuming He -ShanghaiTech University
Yinlin Hu - EPFL
Zeeshan Hayder - Data61